IQVIA: Healthcare data regional compliance complexity requires AI, ML and NLP automation
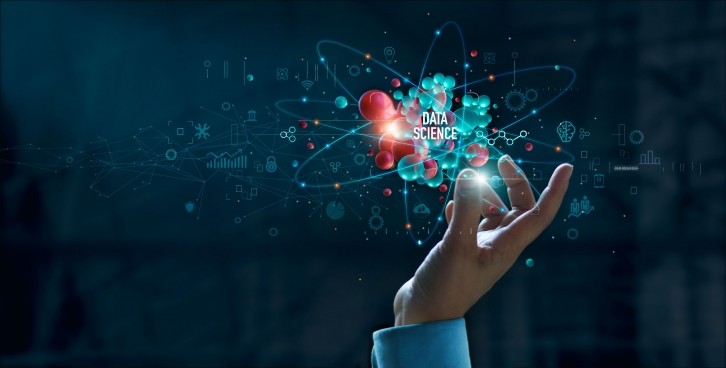
OSP was therefore delighted to speak to expert, Uwe Trinks, practice leader of pharmacovigilance technology at IQVIA about the constantly adapting landscape of healthcare's current data regulatory landscape.
Outsourcing Pharma (OSP): What does healthcare's current data regulatory landscape look like, and how is it changing across regions?
Uwe Trinks (UT): As technology evolves, so automation and use of intelligent agents like AI, Machine Learning (ML) and Natural Language Processing (NLP) is increasing. As a result, new regulations on data use and management are emerging. Organizations across the healthcare spectrum must ensure compliance with these regulations. Early guidance papers focused on devices, but recent ones, like MHRA's "Predetermined Change Control Plans for Machine Learning-Enabled Medical Devices," highlight the need for clear regulations. This focus is evident in FDA's paper on "Using AI & ML in Drug and Biological Product Development" and CIOMS Working Group XIV on "AI in Pharmacovigilance." The power of Generative AI poses challenges to existing legal frameworks, which primarily address human data processing and access.
In the European Union, GDPR aims to empower individuals over their personal data and streamline international business regulations. It includes strict data flow rules, limiting data transfer outside the EU and imposing fines of up to €20 million or 4% of sales for noncompliance. In the US, President Biden signed an Executive Order to implement the EU-U.S. Data Privacy Framework (DPF), known as Privacy Shield 2.0. The July 10, 2023 agreement by the European Commission governs data flow between the US and EU, restricting US Security Services' access to EU data. Contested in the European Court of Justice, organizations hosting data outside Europe must swiftly comply with existing or future agreements. The globalized healthcare supply chain faces increasing challenges in navigating complex data regulations manually and it’s here that automation technologies like AI, ML, and NLP can provide valuable solutions.
OSP: How does GDPR regulate AI?
UT: GDPR regulates AI by mandating transparency in organizations' use of personal data, requiring clear information on AI data processing and the implementation of risk management measures. This transparency is crucial for patient data, especially regarding permitted disclosures to algorithms. For instance, AI can analyze patient data, identify multi-regimen patients, and present de-identified datasets without exposing individuals.
Data minimization, another GDPR requirement, mandates organizations to only collect necessary personal data. In the context of AI's data requirements, compliance can be achieved through techniques like anonymization to reduce processed personal data. GDPR also includes provisions like the right to access, erasure, and data portability relevant to AI. Controlling these rights is simpler in relational databases compared to diverse data lakes, and informed consent documents may need rewriting in the future.Top of FormBottom of Form
OSP: What are the challenges faced by pharma professionals, and how can ML, AI and NLP ease the burden on those working in the pharma industry?
UT: The rising healthcare costs pressure pharma professionals to develop affordable yet effective drugs. Patients seek early, cost-effective treatments amid diseases' increasing complexity. Demand for personalized medicine adds pressure to tailor therapeutics for individual patients. Global and regional regulations complicate and increase costs for drug development. Generics competition forces pharma companies to reduce brand-name drug prices. Challenges include skilled worker shortages, technology adoption, and skill acquisition.
AI, ML, and NLP alleviate pharma professionals' burdens by automating tasks. In drug discovery, these technologies automate screening, toxicity prediction, and drug design, reducing time significantly. In clinical trials, ML automates patient recruitment, data collection, monitoring, and analysis. NLP aids regulatory compliance by automating tasks like reviewing clinical trial data. Generative AI can be a big help in the diagnosis of a disease or in assessing multiple drug regimens for potential interactions or for optimization but both come with its own set of challenges. Intelligent technologies extend to manufacturing, supply chain, documentation creation, and marketing applications, supporting pharma executives in daily tasks.
OSP: How does AI relate to natural language processing?
UT: AI and NLP are interconnected. AI creates intelligent agents for autonomous reasoning, learning, and action. NLP, a subset of AI, focuses on developing systems for understanding and interacting with human language. AI facilitates NLP applications like speech recognition, machine translation, and text summarization. For instance, machine translation systems use AI to translate text between languages, speech recognition systems convert spoken language to text, and text summarization systems generate concise summaries.
In healthcare, NLP finds applications in analyzing vast data from scientific literature, clinical trials, and other sources. It aids in identifying new drug targets, developing candidates, automating regulatory document review, and ensuring compliance.
OSP: How do AI, ML and NLP support safety in a regulatory sense?
UT: Pharma companies require intelligently connected safety, regulatory, and quality processes for precise global operations. AI and NLP play a crucial role in patient safety and adverse event (AE) discovery and reporting post-market.
Automated pharmacovigilance solutions utilize AI and NLP to detect AEs and potential risks in structured and unstructured data from various sources. Web and mobile portals facilitate AE reporting. Soon, systems will autonomously process individual case safety reports from unstructured data, with humans overseeing quality control.
AI, NLP, and automated translation rank safety risks, with NLP providing quick access to safety data from diverse textual sources. Real-time alerts for critical AEs are enabled by NLP's ability to instantly identify related events, enhancing the overall value of AI for users.
OSP: How do AI, ML and NLP help to manage regional healthcare data compliance?
UT: Staying compliant with global and regional healthcare regulations, especially related to data, used to be a complex task requiring extensive coordination. AI simplifies this process, with AI-powered regulatory intelligence platforms automating the identification and monitoring of regulatory changes. By analyzing diverse sources, including government websites and industry publications, AI flags new laws and regulations, assesses critical requirements, and predicts potential impacts. Pharma companies leverage large datasets to understand and comply with specific regulations, such as HIPAA in the US and GDPR in the EU. Automated translation and sharing of documentation centralize processes.
ML-based risk assessment tools identify and assess risks associated with new regulations. NLP-based compliance report generators automatically produce reports for targeted and effective compliance programs.
AI, ML, and NLP provide significant value in monitoring and maintaining regional healthcare data compliance. AI and ML-powered data privacy platforms quickly classify sensitive healthcare data, facilitating comprehensive protection measures. Internally, AI and NLP monitor data access, helping identify compliance violations like unauthorized access. ML-based risk assessment tools assess risks in data processing activities, while NLP-based data auditing tools detect and investigate potential compliance violations in healthcare organizations.
OSP: Which is the best application of AI in the pharma sector?
UT: Determining the best AI application is subjective, considering evolving needs in the pharma sector. However, leveraging AI to expedite drug discovery, utilizing algorithms for identifying effective candidates, stands out. AI-based clinical trial management systems enhance efficiency, bringing therapeutics to market faster for patient access.
Personalized medicine platforms, driven by AI, create customized treatment plans based on individual characteristics, improving outcomes and minimizing side effects. Real-world evidence platforms analyze patient data to glean insights on drug safety and efficacy, enhancing patient care quality.
The potential of AI in the pharma sector is just beginning. As AI evolves and integrates into healthcare, the sector is poised to become more patient-centric.
OSP: There has been an element of resistance to AI, ML and automation in the pharma industry. How can the unconvinced be convinced?
UT: AI and ML adoption signals a profound shift for the cautious pharma industry, given data security concerns and negative perceptions of generative AI. Emphasizing the critical role of "human in the loop," it's vital to recognize AI systems' dependence on training, as wrongly trained systems can produce absurd results. For instance, an image recognition system misidentifying a cow as a camel on the beach due to surrounding sand.
Wholesale change demands understanding AI deployment and implementing safeguards, placing a substantial burden on companies choosing vendors with the requisite skills. Many opt for incremental adoption, testing technologies in a limited scope to realize benefits like enhanced efficiency, reduced costs, improved quality, accelerated drug discovery, and optimized regulatory compliance.
OSP: Where do you see the potential for Generative AI platforms in regulatory compliance?
UT: Generative AI, though early in adoption, holds potential to enhance drug safety through automated case processing, streamlined operations, and improved data access. It identifies trends not easily visible to humans, automates tasks comprehensibly, and provides unique data visualization. Open-source platforms enhance safety insights, yet regulatory concerns persist.
Despite benefits, external Generative AI poses risks, such as false information impacting safety analysis. Transparency challenges and potential misuse by bad actors highlight the need for caution. Mitigating risks involves industry-specific Generative AI solutions, curated training data, and integration with other tools.
IQVIA develops controlled Generative AI for life science, maximizing value through draft reports, signal detection, and trend identification. Implementing Generative AI requires a robust Change Management Framework, recognizing its transformative impact comparable to past industrial revolutions. While rendering some jobs obsolete, it creates new opportunities, marking a significant organizational shift.
OSP: Finally, how does IQVIA help companies stay updated with the ever-changing regulations?
UT: IQVIA empowers companies for proactive regulatory adaptation, ensuring compliance with evolving requirements. Key pillars include:
Regulatory Intelligence: Swift response to changes with real-time global updates from national authorities. Information is disseminated via email, web portals, and mobile apps for up-to-date compliance.
Regulatory Consulting: Services cover gap assessments, compliance program development, and submission support across pharmaceuticals, medical devices, and clinical trials. Expert guidance ensures comprehensive adherence to intricate regulatory demands.
Regulatory Technology: Automated solutions for compliance, offering document management, submission tools, and intelligence platforms. Enhancing efficiency, accuracy, and cost-effectiveness in regulatory processes.